Big Data for Fraud Detection
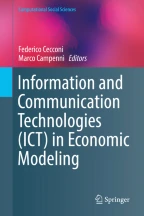
Fraud is domain-specific, and there is no one-solution-fits-all method among fraud detection techniques. To make this chapter more specific and concrete, we provide examples concerning a common type of fraud which is food fraud. Food fraud has irreversible effects since it imposes risks to human life. The aim of this chapter is thus to present a conceptual and methodological solution for real-time fraud detection that can be implemented in the food sector by global food producers, regulatory bodies, or retailers but is generalizable to other domains.
This is a preview of subscription content, log in via an institution to check access.
Access this chapter
Subscribe and save
Springer+ Basic
€32.70 /Month
- Get 10 units per month
- Download Article/Chapter or eBook
- 1 Unit = 1 Article or 1 Chapter
- Cancel anytime
Buy Now
Price includes VAT (France)
eBook EUR 67.40 Price includes VAT (France)
Softcover Book EUR 84.39 Price includes VAT (France)
Hardcover Book EUR 116.04 Price includes VAT (France)
Tax calculation will be finalised at checkout
Purchases are for personal use only
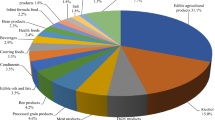
Establishment of a food fraud database and analysis of fraud information based on network data in China
Article 12 January 2022
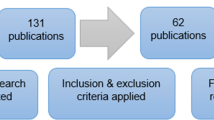
Big data in the food supply chain: a literature review
Article Open access 24 January 2022
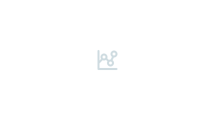
Risky business: food fraud vulnerability assessments
Article Open access 24 September 2022
References
- Barnett, V., & Lewis, T. (1994). Outliers in statistical data. 3rd edition, John Wiley & Sons, Chichester, UK, (pp. 584), ISBN 0-471-93094-6. Google Scholar
- Blakeborough, L., & Giro Correira, S. (2017). The scale and nature of fraud: A review of evidence. ISBN 978-1-78655-682-0 (evidence review undertaken by Home Office Analysis and Insight to bring together what is known about the scale and nature of fraud affecting individuals and businesses in the UK) Google Scholar
- Button, M., Lewis, C., & Tapley, J. (2009). Fraud typologies and the victims of fraud: literature review. London: National Fraud Authority, 40 p. Google Scholar
- Button, M., Lewis, C., & Tapley, J. (2014). Not a victimless crime: The impact of fraud on individual victims and their families. Security Journal, 27(1), 36–54. ArticleGoogle Scholar
- Cabinet Office. (2014). Common areas of spend, Fraud, error and debt, Standard Definition v2.1. Retrieved from http://www.gov.uk/government/uploads/system/uploads/attachment_data/file/340578/CAS-FED-Guidance-version-2.1-July-2014_P1.pdf
- Cerioli, A., & Farcomeni, A. (2011). Error rates for multivariate outlier detection. Computational Statistics & Data Analysis, 55(1), 544–553. ArticleGoogle Scholar
- Chandola, V., Banerjee, A., & Kumar, V. (2009). Anomaly detection: A survey. ACM Computing Surveys (CSUR), 41(3), 15. ArticleGoogle Scholar
- Cressey, D. R. (1950). The criminal violation of financial trust. American Sociological Review, 15(6), 738–743. ArticleGoogle Scholar
- Filzmoser, P., & Hron, K. (2008). Outlier detection for compositional data using robust methods. Mathematical Geosciences, 40(3), 233–248. ArticleGoogle Scholar
- Filzmoser, P., Garrett, R. G., & Reimann, C. (2005). Multivariate outlier detection in exploration geochemistry. Computers & Geosciences, 31(5), 579–587. ArticleGoogle Scholar
- Filzmoser, P., Maronna, R., & Werner, M. (2008). Outlier identification in high dimensions. Computational Statistics & Data Analysis, 52(3), 1694–1711. ArticleGoogle Scholar
- Garrett, R. G. (1989). The chi-square plot: A tool for multivariate outlier recognition. Journal of Geochemical Exploration, 32(1–3), 319–341. ArticleGoogle Scholar
- Gee, J. (2018). The financial cost of fraud. Retrieved from https://www.crowe.com/uk/croweuk/insights/financial-cost-of-fraud-2018
- Gogoi, P., Borah, B., & Bhattacharyya, D. K. (2010). Anomaly detection analysis of intrusion data using supervised & unsupervised approach. Journal of Convergence Information Technology, 5(1), 95–110. ArticleGoogle Scholar
- Guardian, T. (2013). Horsemeat scandal blamed on European meat regulation changes. The Guardian. Retrieved from https://www.theguardian.com/environment/2013/feb/12/horsemeat-scandal-european-regulation-changes
- Hudson, A., Thomas, M., & Brereton, P. (2016). Food incidents: Lessons from the past and anticipating the future. New Food, 19, 35–39. Google Scholar
- Johnson, R. A., & Wichern, D. W. (2002). Applied multivariate statistical analysis (Vol. 5). Upper Saddle River, NJ: Prentice Hall. Google Scholar
- Kassem, R., & Higson, A. (2012). The new fraud triangle model. Journal of Emerging Trends in Economics and Management Sciences, 3(3), 191. Google Scholar
- Lane, T., & Brodley, C. E. (1997). Sequence matching and learning in anomaly detection for computer security. In AAAI Workshop: AI Approaches to Fraud Detection and Risk Management, pp. 43–49. Google Scholar
- Matsumura, E. M., & Tucker, R. R. (1992). Fraud detection: A theoretical foundation. Accounting Review, 753–782. Google Scholar
- Patcha, A., & Park, J.-M. (2007). An overview of anomaly detection techniques: Existing solutions and latest technological trends. Computer Networks, 51(12), 3448–3470. ArticleGoogle Scholar
- R Core Team. (2014). R: A language and environment for statistical computing. Vienna: R Foundation for Statistical Computing. Google Scholar
- Riani, M., Atkinson, A. C., & Cerioli, A. (2009). Finding an unknown number of multivariate outliers. Journal of the Royal Statistical Society: Series B (Statistical Methodology), 71(2), 447–466. ArticleGoogle Scholar
- Rosseeuw, P. J., & Van Zomeren, B. C. (1990). Unmasking multivariate outliers and leverate points. Journal of the American Statistical Association, 85, 633–639. ArticleGoogle Scholar
- Rousseeuw, P. J. (1985). Multivariate estimation with high breakdown point. Mathematical Statistics and Applications, 8, 283–297. ArticleGoogle Scholar
- Spink, J., & Moyer, D. C. (2011). Defining the public health threat of food fraud. Journal of Food Science, 76(9), R157–R163. ArticleGoogle Scholar
- Tennyson, S. (2008). Moral, social, and economic dimensions of insurance claims fraud. Social Research, 1181–1204. Google Scholar
- Wang, C., Viswanathan, K., Choudur, L., Talwar, V., Satterfield, W., & Schwan, K. (2011). Statistical techniques for online anomaly detection in data centers. In 12th IFIP/IEEE International Symposium on Integrated Network Management (IM 2011) and Workshops (pp. 385–392). IEEE. Google Scholar
- Wilks, T. J., & Zimbelman, M. F. (2004). Using game theory and strategic reasoning concepts to prevent and detect fraud. Accounting Horizons, 18(3), 173–184. ArticleGoogle Scholar
Author information
- Avanade Ltd., London, UK Vahid Mojtahed
- Fera Science Ltd., National Agri-food Innovation Campus, Sand Hutton, York, UK Vahid Mojtahed
- Vahid Mojtahed